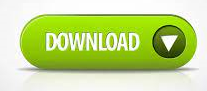
Experimental results show thatĬompared to the state-of-the-art solution Orca, FastServe improves the averageĪnd tail JCT by up to 5.1$\times$ and 6.4$\times$, respectively. We build a system prototype ofįastServe based on NVIDIA FasterTransformer. Memory and host memory for LLM inference. Mechanism that proactively offloads and uploads intermediate states between GPU We design an efficient GPU memory management The higher priority queues than the joined queue are Input length information to assign an appropriate initial queue for eachĪrrival job to join.

Information-agnostic setting of LLM inference, the scheduler leverages the FastServe uses preemptive scheduling to minimize JCT withĪ novel skip-join Multi-Level Feedback Queue scheduler. FastServe exploits theĪutoregressive pattern of LLM inference to enable preemption at the granularity

We present FastServe, aĭistributed inference serving system for LLMs. And my heart begins to condemn me for my past, Feels so hard to let it go. I’ll help you see, help you see, The good that I see in you.

Suffers from head-of-line blocking and long JCT. 2 days ago &0183 &32 He says: Draw close to me, close to me, And I’ll draw close to you. LLM serving systems use run-to-completion processing for inference jobs, which The interactive nature of theseĪpplications demand low job completion time (JCT) for model inference. Download a PDF of the paper titled Fast Distributed Inference Serving for Large Language Models, by Bingyang Wu and 5 other authors Download PDF Abstract: Large language models (LLMs) power a new generation of interactive AIĪpplications exemplified by ChatGPT.
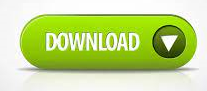